E-Commerce | Natural Language Generation (NLG) | Data-to-Text Automation | Epic Product Descriptions | Auto-Updating Copy | Automated Texts + Product Descriptions | GPT + RPA + DeepL
Deep Dive: Which AI Model Works Best For Product Copy?
Reading Time 10 mins | November 27, 2023 | Written by: Heike Hoppmann
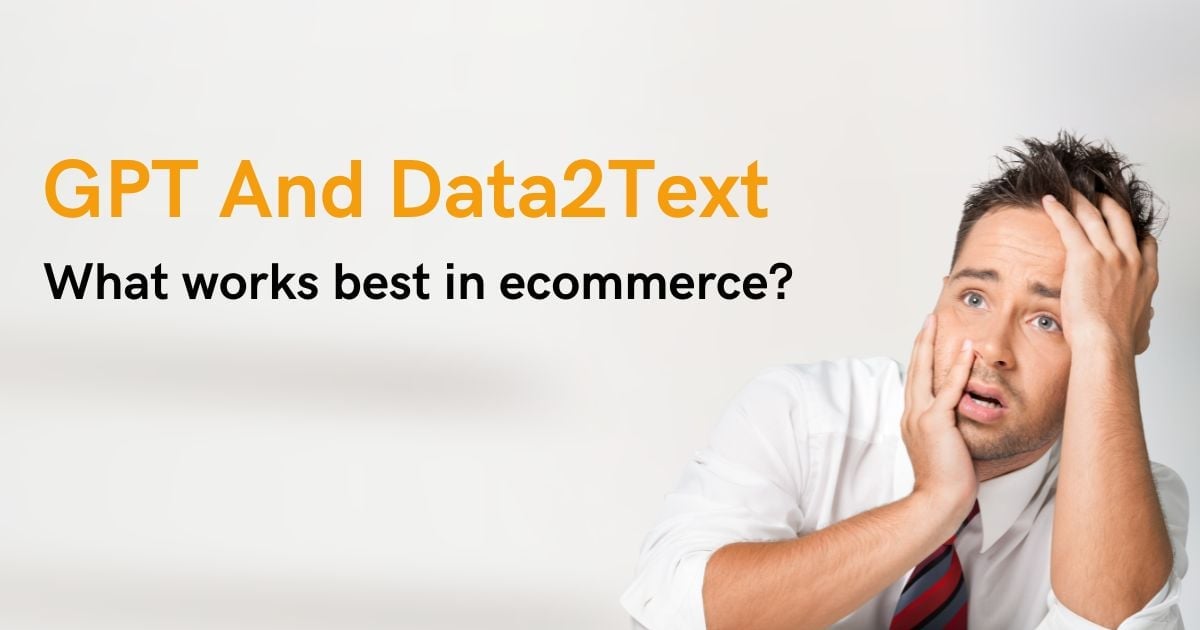
Machine-generated text creation is a relief for copywriters and content managers in e-commerce. Anyone involved in writing texts benefits from it. There are two key technologies: generative AI and rule-based data-to-text systems. Most have already had their first experiences with generative AI, most notably GPT.
But how do these two technologies differ in terms of the challenge of creating effective product descriptions in e-commerce? How can they best support the text challenges in e-commerce?
"The results are always just a prompt away"
Saim Rolf Alkan, CEO AX Semantics
What to expect in this post:
- The two technologies: generative (GPT) and rule-based (AX Semantics)
- How exactly does a generative text AI like GPT work?
- How does rule-based text AI like AX Semantics work?
- The challenges of product descriptions in e-commerce
- How can GPT or AX Semantics help with the challenges of many product descriptions?
- How can GPT be used for product descriptions?
- How can AX Semantics be used for product descriptions?
- Direct comparison of the systems in terms of challenges
- Conclusion
The two technologies: generative (GPT) and rule-based (AX Semantics)
Generative Pre-trained Transformers (GPT) belong to the large language models and are artificial neural networks based on Transformer architecture (a deep learning architecture). They are trained on a large amount of text, meaning they can generate text in natural language without further training.
Data-to-Text refers to the machine-automated production of natural language texts based on data.
How exactly does a generative text AI like GPT work?
Generative AI, GPT, is a language prediction model that calculates probabilities for the next words, similar to word suggestions on a mobile phone.
GPT was trained with texts from the Internet, including Wikipedia, forums, web texts, and book databases. These form the basis of the language prediction model. The system analyzes the language based on the linguistic patterns it recognizes from the training and uses these patterns to create the most likely text option. Since the result is based solely on word probabilities, it often leads to content inaccuracies or nonsensical statements.
How does rule-based text AI like AX Semantics work?
Rule-based text or data-to-text technologies are a subset of artificial intelligence. These programs analyze structured data and generate finished, natural language texts from it.
For rule-based data-to-text programs to work, structured data must be available as a basis. To create finished text from this data, the user configures the rules and logic in the software. This extracts the relevant information from the dataset and translates it into natural language texts. Variances can also be inserted. This means that the structure of a sentence remains the same, but small variables in the sentence change. This generates unique texts again and again, avoiding duplicate content. |
Rule-based or data-to-text software differs from GPT in that it is not just trained with texts from the Internet. Instead, they use data from CRM or PIM systems or stored in Excel, CSV, and JSON files. The data is transferred directly to the software or via an API. In contrast, GPT generates texts completely independently, without further human intervention. The neural network alone produces an output from the given input, which can always bring content inconsistencies. |
The challenges of product descriptions in e-commerce
Product descriptions are essential for an online shop and constitute a large portion of the texts created in e-commerce. However, they come with several tricky challenges:
Quality – the demands of consumers on product descriptions:
Consumers desire sufficient and relevant information about products to make the right purchasing decisions. This may differ in content depending on where consumers are in their customer journey. Additionally, a classification in the current context should not be missing. Topics such as climate change or changed purchasing behavior due to crises influence purchasing decisions. It helps if a product description refers to current developments.
Effectiveness – product descriptions have a job to do:
If a product description meets the expectations of consumers, it naturally has an effect, and this is reflected in the metrics. The task of a content team in e-commerce is to write and optimize product descriptions so that they are effective for the e-commerce company in some way. For example, by contributing to better search engine rankings, increasing conversions, or reducing returns.
Volume – A product description rarely comes alone:
Most online shops have several hundred or more likely several thousand products to present. In some cases, they also have different target markets where the products need to be explained in other languages. This multiplies with the different sales channels, such as the additional sale on Amazon, whose text requirements differ from those of their own shop. So, there is a huge amount of product descriptions to create, possibly translate, and keep up to date.
Processes – multiple roles, many stakeholders, and huge Excel lists:
A look at the editorial processes of product descriptions shows the difficulty of the task (effective) "product descriptions" in e-commerce to be barely adequately addressed.
Process of creating new texts: this process is initiated, for example, when new products are ready for sale. There are tasks to provide information about the product for the copywriters, create a text draft, have it technically reviewed, adjust the text draft, undergo another review, etc. A back-and-forth sending of documents begins, or huge, cumbersome Excel lists with approval stages are created.
Translation process: The supposedly finished product descriptions are sent to various translators of other languages and translated there (hopefully machine-supported). It now requires linguistic and content approval. The translation draft goes back to the translators with comments and so on. An error-prone coordination effort begins.
Update process: New information about products is available. An event changes customers' views on a process. Now it is necessary to adjust existing product descriptions. For thousands of products. Formulate the change, possibly re-approve content, translations… everything starts again. We don't need to go into this challenge further, hardly any online shop manages to meet this task.
What ultimately suffers are the effectiveness of the product descriptions and the content team, always feeling unable to meet the task.
How can GPT or AX Semantics help with the challenges of many product descriptions?
Now it is a blessing that machine-generated texts can help with this task. And certainly, every e-commerce content team that has previously manually written product texts makes its experiences with generative AI, like GPT, to speed up the writing of product texts. Because that is precisely the strength of generative AI: stringing together beautiful words, filling the first words on the white paper. A system optimized for interaction. The pure writing process becomes more creative, in some cases even faster. The challenge lies less in writing but in creating masses of product texts in effective quality, translating and keeping them up to date.
How Can GPT Be Used for Product Descriptions?
Product data can easily be turned into a prompt for (Chat)GPT. In no time, (Chat)GPT drafts several product description drafts, also in different languages. Now an e-commerce company decides for itself whether it accepts possible misinformation, which generative text AIs bring with them due to their technical basis, and puts the drafts online as is or continues to work on the draft basis.
The results – always just a prompt away:
Generative text AI strings words together according to the highest probability. The words sound fantastic, but the devil is in the details. Our example of product descriptions of muslin bedding shows how good-sounding words become useless once nonsensical content or errors creep in – some speak here of hallucinations. In the case of muslin bedding, the texture is significant and an essential characteristic of this material. However, the probability leads to a draft output that contains the sentence: "The fine weave and high-quality cotton ensure an exceptionally soft and smooth surface that gently envelops your skin." Which is simply wrong.
And now? Does the correction of the prompt, the processing of the draft by human hands, begin now? Or does an e-commerce company accept this misinformation? A "post-prompting" often leads to further drafts, which show an inconsistency at another point. For a few product descriptions, this path might still offer quickly accessible support. With many product descriptions, a seemingly fast, simple solution suddenly becomes an endless time sink.
How can AX Semantics be used for product descriptions?
AX Semantics is a rule-based AI or a data-to-text system – optimized for scaling, that is, optimized for handling many repetitive texts. The process with AX Semantics also starts with the product data, which are linked to AX Semantics. Based on the data, the e-commerce content team creates a text concept, in which the content team regulates the desired language, the variance, the words, the phrases, the statements, and framework conditions such as text length. It regulates under what conditions which textual outputs occur. A GPT integration supports the formulation of phrases and variants of the text concept and makes suggestions without tedious prompting. At a point where creative input and draft formulations help the writer or author. Every word, every sentence, every phrase is approved by the author before text production. So, in the end, only what the content team has previously defined is in the product description.
In the context of challenges in e-commerce, this means that a unique text conception and approval process is required for a vast number of product descriptions. As soon as the context changes for consumers or other occasions arise that necessitate adjustments to the product texts, these modifications can be applied to all product descriptions at the push of a button, either through an existing rule or one that is added. The text concept, rather than the individual product description, is translated, or more accurately, localized. As a result, the translation also takes place centrally and therefore before the production of texts in different languages. Translators work in an environment optimized for translation and are supported by a DeepL integration during the translation process.
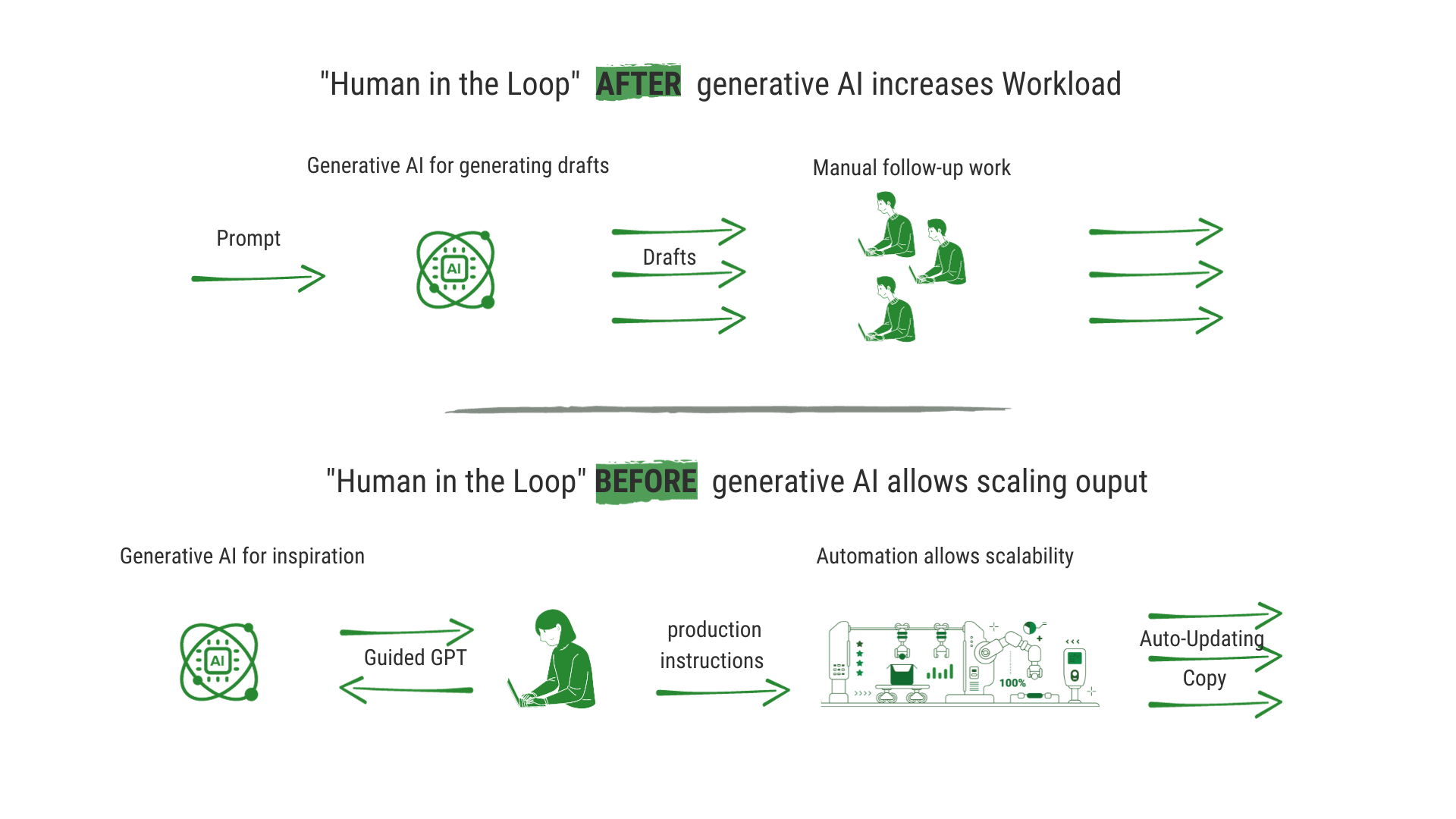
The direct comparison of the systems with respect to the challenges in product descriptions.
Challenges | AX Semantics | GPT |
Quality | + Enables updates and optimizations at the push of a button + Clarity, understandability, relevance, and accuracy are under the author's control Integrated GPT and DeepL support in the creative process |
+ Quickly produces linguistically appealing texts - Improvements in drafts are cumbersome - Updates need to be manually initiated and organized |
Effectiveness | + Optimizations can be made at any time at the push of a button | - Optimizing existing product texts is cumbersome |
Volume | + Product descriptions are easy to "manage" because thousands of product texts can be created, translated, and updated through central rules | - Drafts cannot be published unseen, necessitating further work on the drafts |
Processes | + Automated workflows reduce editorial processes + Integrated GPT and DeepL support the processes |
+Writing process can be partially faster - Approval, translation, and updating processes still need to be outsourced |
Conclusion:
Both generative text AI and rule-based, data-to-text systems have their place as AI-supported text generation technologies. Each technology assists in its own specific way. With regard to the specific challenges of product descriptions in e-commerce, generative text AI alone is not necessarily a relief. Especially with a large number of product descriptions, a rule-based system is more supportive in managing the bulk of texts and meeting the requirements of these texts.
Further information on data2text automated text generation.
AI text tools are based either on GPT or Data-to-Text technology. To use Data-to-Text software, you need structured data to create high-quality and relevant content. Your data is converted into text, ensuring consistency and uniform quality. You can configure the texts according to your preferences. AI writing tools based on GPT are trained with hundreds of billions of words representing a significant portion of the internet. GPT tools are primarily suitable as a source of ideas and aids for creating individual texts, like blog posts.
Natural Language Generation (NLG) refers to the automated generation of natural language by a machine. As a subfield of computational linguistics, content generation is a special form of artificial intelligence. The generation of natural language is used in many industries and for many purposes, such as in e-commerce, financial services, and the pharmaceutical industry. It is considered particularly effective for automating repetitive and time-consuming writing tasks like product descriptions, reports, or personalized content.
Data-to-Text is used in areas such as e-commerce, finance, pharmaceuticals, media, and publishing. GPT can be helpful in brainstorming and finding inspiration, for example, when the user suffers from writer's block. Using GPT in chatbots to answer recurring customer queries is also very useful, as it is inefficient and impractical to have these texts created by humans. More information can be found at our Data2text section.
Heike Hoppmann
Heike Hoppmann believes in the power of words. The use of words can be creative, but above all it must be purposeful. Writing is not an end in itself. As a mentor and coach for editorial management and digital transformation processes, she has successfully conveyed this idea to many online editors. And today it is the guiding principle of her work as PR Manager at AX Semantics GmbH.